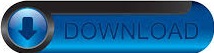
In machine learning, we commonly use the statistical framework of maximum likelihood estimation as a basis for model construction.
SPARSE CATEGORICAL CROSS ENTROPY UPDATE
To understand how the gradients are calculated and used to update the weights, refer to my post on backpropagation with gradient descent.Ī machine learning model such as a neural network attempts to learn the probability distribution underlying the given data observations. The average over all losses constitutes the cost. From the loss function, we can derive the gradients which are used to update the weights. The loss function in a neural network quantifies the difference between the expected outcome and the outcome produced by the machine learning model. If the shape of sample_weight is invalid.This post introduces the most common loss functions used in deep learning. (Note dN-1 because all loss functions reduce by 1 dimension, usually axis=-1.) If reduction is NONE, this has shape otherwise, it is scalar. (Note on dN-1: all loss functions reduce by 1 dimension, usually axis=-1.) If the shape of sample_weight is (or can be broadcasted to this shape), then each loss element of y_pred is scaled by the corresponding value of sample_weight. If sample_weight is a tensor of size, then the total loss for each sample of the batch is rescaled by the corresponding element in the sample_weight vector. If a scalar is provided, then the loss is simply scaled by the given value. Optional sample_weight acts as a coefficient for the loss. shape =, except sparse loss functions such as sparse categorical crossentropy where shape = Returns the config dictionary for a Loss instance. View source a Loss from its config (output of get_config()). Defaults to 'sparse_categorical_crossentropy'. Please see this custom training tutorial for more details. When used with tf.distribute.Strategy, outside of built-in training loops such as tf.keras compile and fit, using AUTO or SUM_OVER_BATCH_SIZE will raise an error. For almost all cases this defaults to SUM_OVER_BATCH_SIZE. AUTO indicates that the reduction option will be determined by the usage context. **Note - Using from_logits=True may be more numerically stable. By default, we assume that y_pred encodes a probability distribution. Whether y_pred is expected to be a logits tensor. Usage with the compile() API: pile(optimizer='sgd', Scce(y_true, y_pred, sample_weight=tf.constant()).numpy() # Using 'auto'/'sum_over_batch_size' reduction type. The shape of y_true is and the shape of y_pred is. In the snippet below, there is a single floating point value per example for y_true and # classes floating pointing values per example for y_pred. There should be # classes floating point values per feature for y_pred and a single floating point value per feature for y_true. If you want to provide labels using one-hot representation, please use CategoricalCrossentropy loss. We expect labels to be provided as integers. Use this crossentropy loss function when there are two or more label classes. Tf.compat.v1. tf.(įrom_logits=False, reduction=losses_, Tf.losses.SparseCategoricalCrossentropy Compat aliases for migration Tf. View source on GitHubĬomputes the crossentropy loss between the labels and predictions.
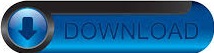